H3ABioNet provides guidelines on the use of clinical data, data management and data standardization
Data & Standards
FAIR Data
- Training materials
- Software and workflows
- Data
What is FAIR Data?
These are data which meet the principles of FAIR, that is, Findable, Accessible, Interoperable and Reusable. These principles are further explained as follows:
FindableData and Metadata should be easy to find for both humans and computers. This is classified as an essential component of what is called the FAIRification process.
F1. (Meta)data are assigned a globally unique and persistent identifier
F2. Data are described with rich metadata (defined by R1 below)
F3. Metadata clearly and explicitly include the identifier of the data they describe
F4. (Meta)data are registered or indexed in a searchable resource
(Ref: https://www.go-fair.org/fair-principles/)
AccessibleOnce the required data has been found, the user needs to have the knowledge of the data accessibility which also likely includes its authentication and authorisation.
A1. (Meta)data are retrievable by their identifier using a standardised communications protocol
A1.1 The protocol is open, free, and universally implementable
A1.2 The protocol allows for an authentication and authorisation procedure, where necessary
A2. Metadata are accessible, even when the data are no longer available
(Ref: https://www.go-fair.org/fair-principles/)
InteroperableIn this aspect, data need to be merged/integrated other data in conjunction with applications or workflows for data analysis, storage, and processing.
I1. (Meta)data use a formal, accessible, shared, and broadly applicable language for knowledge representation.
I2. (Meta)data use vocabularies that follow FAIR principles
I3. (Meta)data include qualified references to other (meta)data
(Ref: https://www.go-fair.org/fair-principles/)
ReusableThe main goal of FAIR is to get the most out of the re-usability of data. To achieve this goal, retrieved data should be well-described for easy manipulation into different settings.
R1. Meta(data) are richly described with a plurality of accurate and relevant attributes
R1.1. (Meta)data are released with a clear and accessible data usage license
R1.2. (Meta)data are associated with detailed provenance
R1.3. (Meta)data meet domain-relevant community standards
(Ref: https://www.go-fair.org/fair-principles/)
Data FAIRifcation process
The data FAIRifcation process is made up of the following:
- Non-FAIR dataset retrieval
- Retrieved dataset analysis
- Define the semantic model
- Make dataset linkable
- Assign license
- Metadata definition for the dataset
- Publish FAIR data resource.
FAIR tools:
- FAIRifier: This tool is designed to address the commonly encountered problems and data-manipulation tasks in the FAIRification process. It allows the user to mash together data and metadata, data license, the data model, and the chosen ontologies and identifiers. It also allows users to directly publish data on a FAIR Data Point (FDP). (Ref: https://github.com/FAIRDataTeam/FAIRifier)
- FAIR Data Point (FDP): This tool allows data owners to publish meta(data) in a FAIR manner. It also offers a graphical user interface (GUI) for human clients and an application programming interface (API) for software clients. (Ref: https://github.com/FAIRDataTeam/FAIRDataPoint-Spec)
- Metadata Editor: This tool makes it easy for non-technical users to define and publish the metadata required by a FAIR Data Point (FDP).
- FAIR Search Engine: This tool finds the metadata available on FAIR Data Points or compatible data repositories, indexes them, and provides a search interface.
- ORKA (The Open, Reusable Knowledge graph Annotator): This tool supports easy human curation of knowledge graphs by offering service including graph annotation and capturing the provenance of the annotator and the original statement.
The diagram below provides information on how we will be helping to make H3Africa data FAIR:
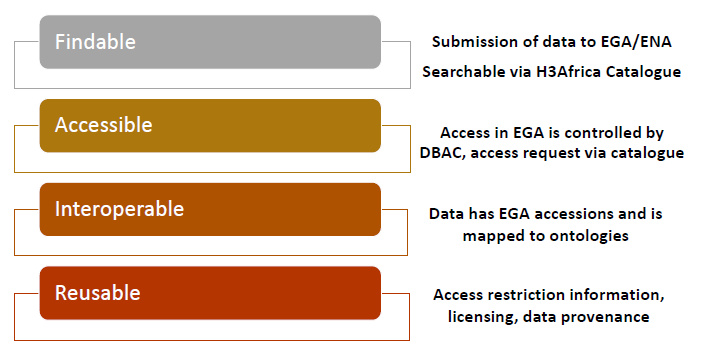